**July 31, 2023 – Bridging the Language Gap in AI: Equal Opportunities for Users Worldwide**
In the realm of artificial intelligence, the language used by users has a significant impact on the cost of utilizing large language models (LLMs), potentially leading to an AI divide between English speakers and speakers of other languages. Recent research has shed light on the fact that due to the cost measurement and billing methods adopted by services like OpenAI, the expenses associated with English input and output are considerably lower than those of other languages. For instance, processing Simplified Chinese comes at approximately twice the cost of English, while Spanish incurs 1.5 times the expense, and Shan, a language spoken in Myanmar, requires a staggering 15 times the cost of processing English content.
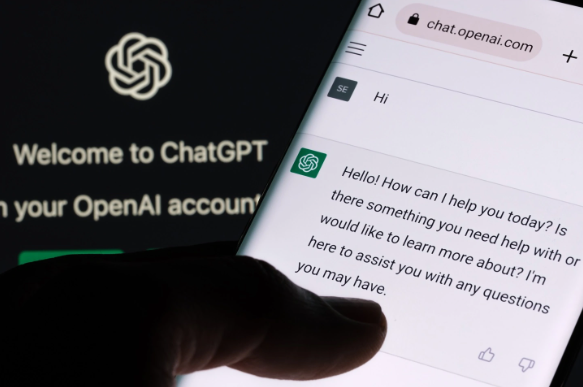
A thought-provoking study conducted by Oxford University and shared by Twitter user Dylan Patel (@dlan522p) reveals that instructing an LLM to process a sentence in Shan consumes 198 tokens, whereas the same sentence in English only requires 17 tokens. Tokens represent the computational cost of accessing an LLM through APIs like OpenAI’s ChatGPT or Anthropic’s Claude 2. Consequently, it becomes evident that processing Shan sentences using such services costs 11 times more than processing English sentences.
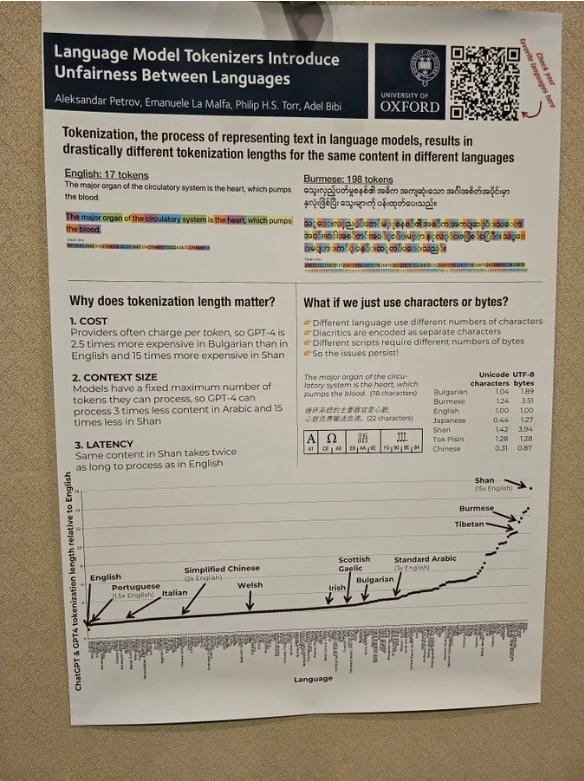
This discrepancy arises from the tokenization model adopted by artificial intelligence companies, where user input is converted into computational costs. Languages other than English, like Chinese, exhibit distinct and more intricate structures, both in terms of grammar and character count, leading to a higher tokenization rate. For instance, based on OpenAI’s GPT3 tokenizer, the phrase “你的爱意” (your affection) requires only two tokens in English but eight tokens in Simplified Chinese. Despite the Chinese text consisting of only 4 characters (你的爱意), the English counterpart contains 14 characters.
Addressing this language-dependent cost difference is crucial for achieving a more equitable and inclusive AI landscape worldwide. By exploring avenues to reduce the computational expenses associated with processing languages other than English and implementing fair pricing strategies, AI companies and research institutions can pave the way for equal opportunities and accessibility for users of all languages. Bridging the language gap in AI is not merely about translation; it requires a fundamental transformation in how we approach language processing and democratize the benefits of AI for people from diverse linguistic backgrounds.