October 21, 2024 – Recent reports indicate that NVIDIA’s latest research could potentially revolutionize the future of artificial intelligence. The company’s research team has introduced a novel neural network architecture called the Normalized Transformer (nGPT).
This innovative architecture performs representation learning on a hypersphere, resulting in a remarkable increase in the training speed of large language models (LLMs) while maintaining model accuracy. According to the reports, the nGPT architecture can achieve up to a 20-fold improvement in training speed.
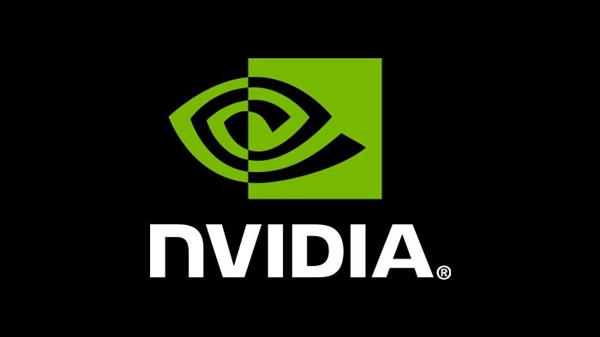
The key aspect of the nGPT architecture lies in the normalization of all vectors, including embeddings, multilayer perceptrons (MLPs), attention matrices, and hidden states, to unit norm. This normalization process allows input tokens to move across the surface of the hypersphere, with each layer of the model contributing to the final output prediction through displacements.
Experimental results demonstrate that the nGPT requires significantly fewer training steps compared to standard Transformer models. Specifically, the reduction ranges from 4 to 20 times, depending on the sequence length. For instance, in a 1k context, the training speed is enhanced by a factor of 4; in a 4k context, it improves by 10 times; and in an 8k context, the speedup is as much as 20 times.
Researchers explain that the optimization path of the nGPT begins with points on the hypersphere and involves displacements to contribute to the final output prediction. These displacements are defined by the MLP and attention modules within the architecture. This approach not only enhances training speed but also improves the stability of the model, paving the way for potential advancements in the field of AI.