May 22 2025 – A recent study conducted by the Massachusetts Institute of Technology (MIT) has unveiled significant shortcomings in artificial intelligence (AI)’s comprehension of negation terms like “no” and “not,” posing potential severe risks, especially in critical fields such as healthcare.
Despite the rapid advancement of AI, which now boasts practical capabilities ranging from diagnosing illnesses and composing poetry to autonomous driving, the technology still struggles with interpreting negative expressions.
Led by PhD candidate Kumail Alhamoud, a collaborative team from MIT, OpenAI, and the University of Oxford discovered that leading AI models, including ChatGPT, Gemini, and Llama, frequently default to affirmative interpretations when processing negated statements, thereby disregarding the negative semantics.
The research report underscores that the potential dangers of this phenomenon are particularly pronounced in medical settings. For instance, an AI system might misinterpret statements like “no fracture” or “not enlarged,” leading to dire consequences.
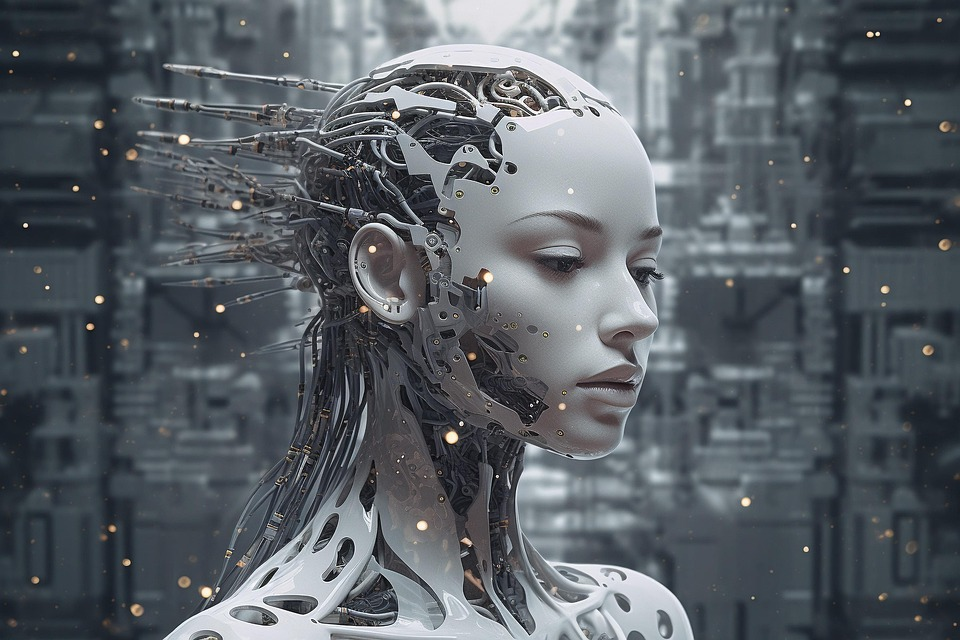
Drawing from a blog post, the root cause of the issue is not attributed to a lack of data but rather to the training methodology employed for AI. Kian Katanforoosh, an adjunct professor of deep learning at Stanford University, highlights that most language models rely on pattern prediction rather than logical reasoning.
Consequently, when faced with phrases like “not good,” AI may still infer a positive sentiment due to the presence of the word “good.” Experts emphasize that unless models are equipped with logical reasoning capabilities, similar subtle yet catastrophic errors will persist.
Franklin Delehelle, the chief research engineer at Lagrange Labs, also notes that while AI excels at mimicking patterns found in training data, it lacks the ability to innovate or handle scenarios outside its training scope.
The research team has made initial strides in enhancing model performance by incorporating synthetic negation data. However, mastering fine-grained nuances in negation remains a formidable challenge.
Katanforoosh cautions that AI’s misinterpretation of negation is not merely a technical flaw but could precipitate critical errors in sectors such as law, healthcare, and human resources. He advocates that the solution lies not in amassing more data but in integrating statistical learning with structured thinking to bolster the logical prowess of models.