May 14 2025 – Tech publication The Decoder reported yesterday that Jakub Pachocki, OpenAI’s senior leader in advanced model development, highlighted the emerging potential of AI reasoning models to autonomously generate knowledge. Unlike human cognition, Pachocki emphasized that this reasoning process stems from a data-driven, algorithm-based framework rather than mimicking human thought patterns.
According to the post, Pachocki outlined a two-stage learning process for AI. The first phase involves unsupervised pre-training, where models ingest vast datasets to construct an unconscious, timeline-free “world model” that captures foundational structures of reality. The second stage transforms this base model into a practical assistant through reinforcement learning combined with human feedback (RLHF). Pachocki underscored the critical role of this phase, particularly in the latest reasoning models.
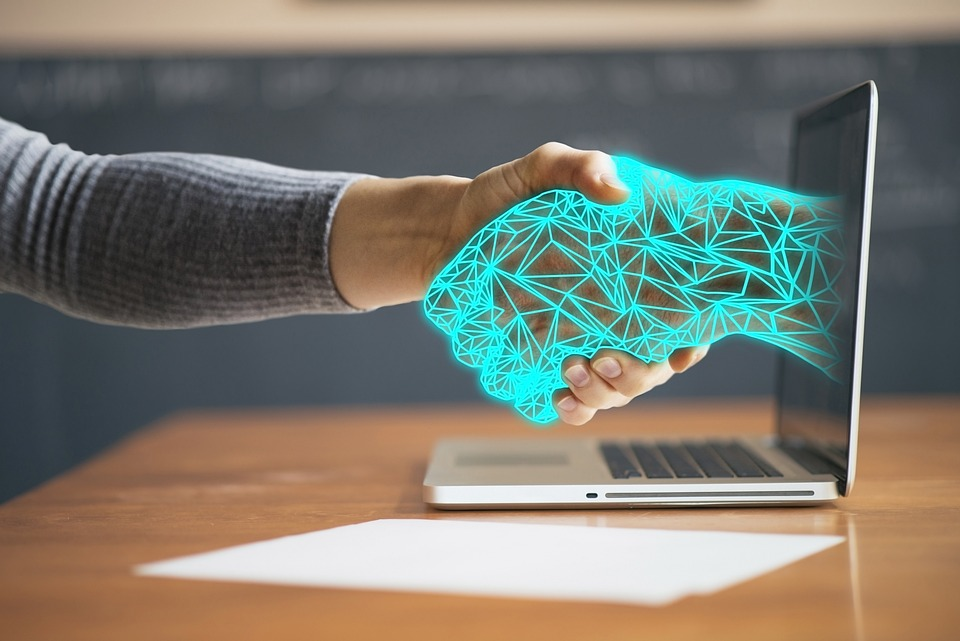
While OpenAI employs traditional reinforcement learning for tasks with clear right-or-wrong metrics, RLHF is favored for addressing complex, nuanced challenges—despite its scalability limitations. Pachocki also questioned whether pre-training and reinforcement learning should remain distinct phases, arguing that reasoning models’ “thought processes” are inherently tied to pre-training data, necessitating deeper integration between the two.
A recent study aligns with Pachocki’s perspective, suggesting that reasoning training doesn’t introduce entirely new capabilities but enhances how models apply existing knowledge. For instance, models can tackle familiar problems more systematically. Pachocki agreed, adding that models have demonstrated the capacity to uncover novel insights, laying groundwork for future AI applications.
Regarding artificial general intelligence (AGI), Pachocki acknowledged his evolving views. He recalled viewing AI mastering Go as an unattainable goal during his student years—a belief shattered by AlphaGo’s 2016 victory. Today, he prioritizes AI’s economic impact as the next frontier, stressing the need for AI to deliver tangible business outcomes and pursue autonomous research. He forecasts “meaningful progress” in AI-driven autonomous research by the end of the decade, predicting even quasi-autonomous software development systems could emerge this year.